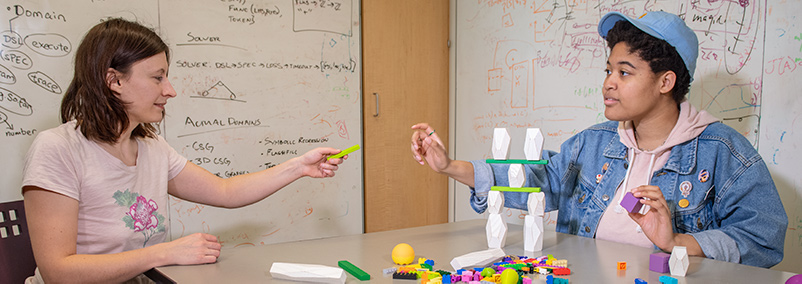
The goal of this project is to model how do humans so quickly and accurately infer goals and anticipate actions of other agents they may encounter in everyday life, such as humans, animals, or robots. We approach this endeavor by building a computational inverse-planning model, which we test using psychophysical experimental tasks. In our experiments human participants view videos of actors carrying out various tasks, and respond by inferring the actor's goal before the task is completed. For example, participants view actors reaching to pick up one of many objects placed table and predict the intended object before it is picked up. Our goal is to accurately model human goal predictions across a variety of tasks and physical scenes, with actors assuming different body poses, and while navigating physical obstacles to complete their goal. Our model incorporates sophisticated cognitive models of human action planning and navigation, inverse Bayesian inference, and Theory Of Mind, which enables it to make predictions quickly and with very little training.
Humans perceive the world in rich social detail. In just a fraction of a second, we not only detect the objects and agents in our environment, but quickly recognize agents interacting with each other. The ability to perceive and understand others’ social interactions develops early in infancy and is shared with other primates. Recently, we identified a region in the human posterior STS that represents both the presence and nature (help vs. hinder) of social interactions. However, the neural computations carried out in this region are still largely unknown. We propose comparing two different types of computational models (feedforward CNNs and generative models) to address this question.
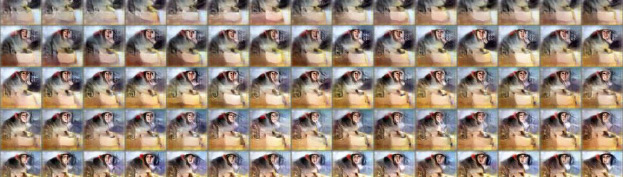
One key problem in both primate and machine visual object recognition is the issue of representation. Given the practical infinity of visual objects and scenes in the world, and the relatively few neurons tasked to represent them, what shapes or features should any given neuron learn to encode to be most efficient? In collaboration with other members of CBMM (Drs. Livingstone and Kreiman), we have been working to identify the set of representations encoded in the primate brain using generative adversarial networks. This novel approach has revealed that neurons encode rich scenes comprising shapes, colors and textures. In this project, we will focus on the issue of invariance, defining the types of representations that facilitate robustness in the face of contextual variations such as size, position and illumination.
The aim of this project is to study the role of the thalamus and sleep oscillations in the flexible consolidation of episodic memories. For this, we will characterize, at the cellular and population level, the dynamics of thalamocortical activity in relation to hippocampal memory reactivation during the oscillations that characterize non-REM sleep (slow oscillation and spindles). We will test specific hypotheses about the millisecond coordination of single thalamic cells with hippocampal representations of episodic memory during sleep spindles, an oscillation that is thought to facilitate the integration of memory acquired by hippocampal networks into generalized representations in the neocortex.
People’s ability to understand other people’s actions, predict what they’ll do next, and determine if they are friends or foes, relies on our ability to infer their mental states. This capacity, known as Theory of Mind, is a hallmark of human cognition: it is uniquely human, it involves reasoning about agents interacting with objects in space, and it requires interpreting observable data (namely, people’s actions) by appealing to unobservable concepts (beliefs, and desires). As part of CBMM, I have developed computational models that capture with quantitative accuracy how we infer other people’s competence and motivation, how we determine who is knowledgeable and who is ignorant, how we predict what people might do next, and how we decide if they’re nice or mean [1]. While these models mimic the computations behind mental state reasoning, their implementations rely on biologically implausible architectures and have a high computational overhead due to the sampling-based approach to inference. This project consists of two parallel studies. The first focuses on studying how visual search supports rapid goal recognition. The second combines probabilistic programming with deep neural networks [2] to develop faster Theory of Mind models that can be used to generate testable neural hypotheses about how Theory of Mind is implemented in the brain.