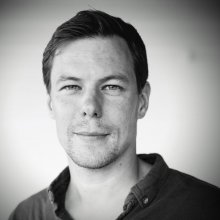
Thomas Serre, Cognitive, Linguistic & Psychological Sciences Department, Carney Institute for Brain Science, Brown University
Title: Feedforward and feedback processes in visual recognition
Abstract: Progress in deep learning has spawned great successes in many engineering applications. As a prime example, convolutional neural networks, a type of feedforward neural networks, are now approaching – and sometimes even surpassing – human accuracy on a variety of visual recognition tasks. In this talk, however, I will show that these neural networks and their recent extensions exhibit a limited ability to solve seemingly simple visual reasoning problems involving incremental grouping, similarity and spatial relation judgments. Our group has developed a recurrent network model of classical and extra-classical receptive fields that is constrained by the anatomy and physiology of the visual cortex. The model was shown to account for diverse visual illusions providing computational evidence for a novel canonical circuit that is shared across visual modalities. I will show that this computational neuroscience model can be turned into a modern end-to-end trainable deep recurrent network architecture which addresses some of the shortcomings exhibited by state-of-the-art feedforward networks for solving complex visual reasoning tasks. This suggests that neuroscience may contribute powerful new ideas and approaches to computer science and artificial intelligence.
Details
43 Vassar Street, Cambridge MA 02139