We will use a set of questions on images to measure performance of our computational models.
The projects that cuts best across all the thrusts consist of an open set of questions on a set of databases of images and videos. The questions are designed to measure intelligent understanding of the physical and of the social world by our models. We will use the performance on these questions to measure our progress during the initial five years of the Center. The overall challenge includes questions such as:
- what is there?
- who is there?
- what is the person doing?
- who is doing what to whom?
- what happens next?
Performance of a model is assessed by evaluating a) how similarly to humans our neural models of the brain answer the questions, and b) how well their implied physiology correlates with human and primate data obtained by using the same stimuli.
Our Turing++ questions require more than a good imitation of human behavior; computer models should also be human-like at the level of the implied physiology and development. Thus the CBMM test of models uses Turing-like questions to check for human-like performance/behavior, human-like physiology, and human-like development. Because we aim to understanding the brain and the mind and to replicate human intelligence, the challenge intrinsic to the testing is not to achieve best absolute performance, but performance that correlates strongly with human intelligence measured in terms of behavior and physiology. We will compare models and theories with fMRI and MEG recordings, and will use data from the latter to inform our models. Physiological recordings in human patients and monkeys will allow us to probe neural circuitry during some of the tests at the level of individual neurons. We will carry out some of the tests in babies to study the development of intelligence.
The series of tests is open-ended; we will rely on a set of databases and add to them during the next five years. The initial ones, e.g. face identification, are tasks that computers are beginning to do and where we can begin to develop models and theories of how the brain performs the task. The later ones, e.g. generating stories explaining what may have been going on in the videos and answering questions about previous answers, are goals for the next five years of the Center and beyond.
The Research Thrusts and the core CBMM challenges: Social Intelligence (Research Thrust 4) is responsible for measuring human performance and mapping the brain areas involved. Circuits for Intelligence (Thrust 2) is devoted to neural circuits, and Development of Intelligence (Thrust 1) is designed to map the development of perception/language abilities and model the interplay of genes and experience. The theory platform, Enabling Theory (Thrust 5), will connect all these levels and inform the algorithms that will be implemented within Vision and Language (Thrust 3), which will take the lead on the engineering side. The modeling and algorithm development will be guided by scientific concerns, incorporating constraints and findings from our work in cognitive development, human cognitive neuroscience, and systems neuroscience. Each Research Thrust is expected to contribute to the development of these models and algorithms and will be evaluated at the appropriate level of its contribution. For instance, the challenge of the development thrust is to create systems that represent objects the way a 3-month-old baby does; an analogous challenge in the neural circuit thrust is to develop models and theories that fit the physiological data, e.g. some part of the ventral stream. These efforts likely would not produce the most effective AI programs today (measuring success against objectively correct performance); the core assumption behind this challenge is that by developing such programs and letting them learn and interact, we will get systems that are ultimately intelligent at the human level.
Collaborative projects in the Center will have to pass the litmus test of contributing directly to demonstrable progress as measured by the "CBMM challenge."
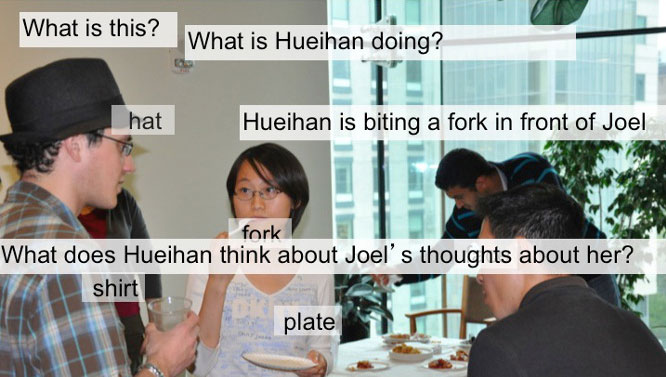
What does understanding a scene mean? For now just notice that this shows understanding of what objects are there and where they are — so the physical world — but also understanding what people do and the interaction between them — the social world.
Computer vision can now do quite a bit of the what and where questions but very little of the rest…
we are still far from image understanding.