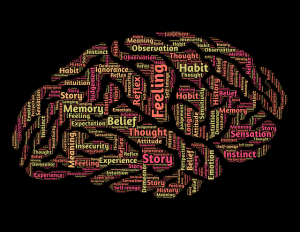
AAAI Spring Symposium Series
Science of Intelligence:
Computational Principles of Natural and Artificial Intelligence
Organized by the Center for Brains, Minds and Machines
Dates: March 27 - 29 2017
Place: Stanford University, Palo Alto, CA - room 002 History Building
Overview
Understanding intelligence -how we may be able to replicate intelligence in machines, and how the brain produces intelligent behavior- is one of the greatest challenges in science and technology.
There are many aspects of human intelligence which have been impossible so far to replicate in artificial intelligent systems. As a trivial example, humans need a remarkably small amount of training to learn to perform a new patter recognition task compared to state-of-the-art artificial intelligence systems
The Science of Intelligence is a new emerging field dedicated to developing a computation-based understanding of intelligence -both natural and artificial- and to establishing an engineering practice based on that understanding. This symposium is designed to bring together experts in artificial intelligence, cognitive science, and computational neuroscience to share and discuss the advances and the challenges in the scientific study of natural and artificial intelligence.
The participants are expected to discuss how intelligence works at a computational level, how intelligence is grounded in neural and silicon hardware, how it develops in early life, and how it is used for social interaction. The symposium will emphasize differences and similarities between natural and artificial intelligence, with the goal of making explicit common computational principles of natural.
Keynote Speakers
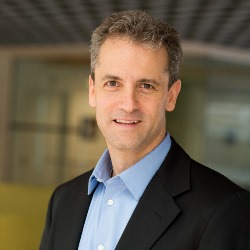
(MIT)
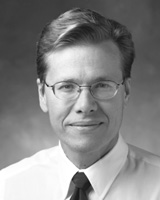
(Stanford)
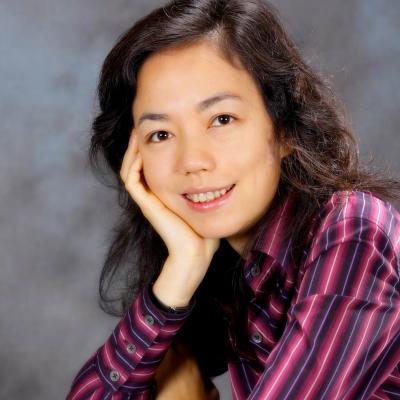
(Stanford)
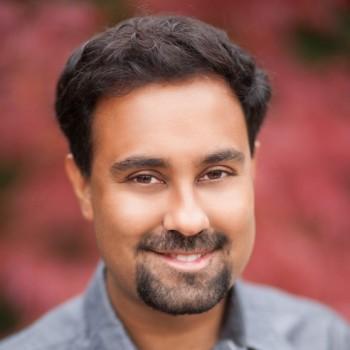
(Stanford)
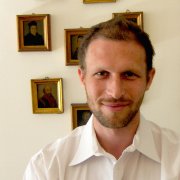
(Harvard)

(University of Texas at Austin)

(Harvard)

(Institute for the Study of Learning and Expertise)

(TTI-Chicago)
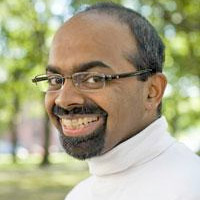
(Harvard)
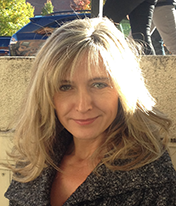
(MIT)

(Caltech)

(MIT)
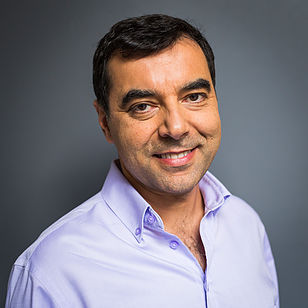
(Hebrew University and Mobileye)
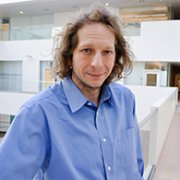
(MIT)
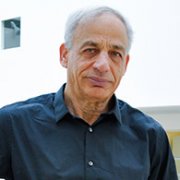
(Weizmann Institute)
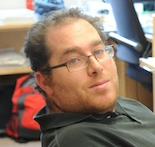
(Stanford)
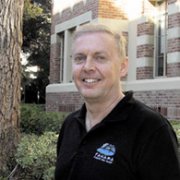
(Johns Hopkins)
Call for Participation
This will be a 3-day symposium consisting on keynote talks, oral and poster presentations, panel discussions and a doctoral consortium.
DEADLINE ABSTRACT SUBMISSION: October 28, 2016 at 11:59pm (UTC-12)
REGISTRATION: Registration is available at the AAAI registration website.
Students that submitted an abstract will be eligible for the doctoral consortium. Selected students will be assigned a mentor from the keynote speakers to discuss their work and future career plans.
Topics
The goal of the symposium is to bring together experts from neuroscience and engineering who study the computational principles of intelligence in brains and in machines. The list of the topics of interest of the symposium is multidisciplinary:
■ Cognitive science
■ Computational neuroscience
■ Probabilistic modelling and inference of behaviour
■ Computational Vision
■ Computational Linguistics
■ Machine Learning
■ Neural Networks
■ Statistical Learning Theory
■ Computer Vision
■ Speech Recognition
Schedule
|
Mon, March 27
|
Tue, March 28
|
Wed, March 29
|
9:00 - 9:45
|
S. Ullman
|
J. Tenenbaum
|
S. Ganguli
|
9:45 - 10:30
|
P. Perona
|
Fei-Fei Li
|
L. Mahadevan
|
10:30 - 11:00
|
Break
|
Break
|
Break
|
11:00 - 11:45
|
S. Gershman
|
A. Oliva
|
Panel: Oliva, Yamins,
Donoho, Poggio |
11:45 - 12:30
|
K. Grauman
|
D. Yamins
|
|
12:30 - 13:15
|
Lunch
|
Lunch
|
Lunch
|
13:15 - 14:00
|
|||
14:00 - 14:45
|
J. DiCarlo
|
G. Kreiman
|
|
14:45 - 15:30
|
K. Livescu
|
P. Langley
|
|
15:30 - 16:00
|
Break
|
Break
|
|
16:00 - 16:45
|
Posters session
|
A.Shashua
|
|
16:45 - 17:30
|
A. Yuille
|
||
17:30 - 18:00 | Break | Break | |
18:00 - 19:00
|
Reception
|
Plenary - Poggio
|
|
Keynote Talks Description and Slides
Shimon Ullman (Monday 9:00 - 9:45)
Digital baby: unguided learning of complex visual tasks
Humans learn to perceive and understand the world in a fast and unsupervised manner. Already early in development, infants learn without guidance to solve visual problems that are highly challenging for computational methods. Striking examples of tasks, which are among the earliest to be learned, are learning to recognize hands, learning to follow other peoples’ direction of gaze, perform segmentation, and understand spatial relations such as ‘containment’. I will describe a model that can imitate infants learning of these concepts. The model is shown a stream of natural videos, and it learns without any supervision to segregate objects, extract meaningful spatial relations between them, and detect human hands as well as gaze direction, in complex natural scenes. The key to the successful learning comes from the use by the system of internal teaching signals, which guide the learning process along a path that leads it to acquire meaningful representations and sophisticated detection processes.
Pietro Perona (Monday 9:45 - 10:30)
Towards a computational approach to behavior - [slides]
The brain's main job is to control behavior. In order to understand the brain we need to be able to measure and understand behavior and this will require a computational understanding of what behavior means. I will describe our efforts in building automated systems to measure and analyze the trajectories, actions and activities of animal models such as fruit fly Drosophila and mouse. I will speculate on future directions including establishing causal links between brain activity and behavior.
Sam Gershman (Monday 11:00 - 11:45)
Where do hypotheses come from? - [slides]
Why are human inferences sometimes remarkably close to the Bayesian ideal and other times systematically biased? Some deviations may arise from algorithmic processes approximating Bayes' rule. In one version of this account, hypotheses are generated stochastically from a sampling process. While this approximation will converge to the true posterior in the limit of infinite samples, humans may generate a small number of samples due to time pressure and cognitive resource constraints. This theory can synthesize a wide range of disparate observations about human reasoning.
Kristen Grauman (Monday 11:45 - 12:30)
Learning How to Move and Where to Look from Unlabeled Video - [slides]
The status quo in visual recognition is to learn from batches of unrelated Web photos labeled by human annotators. Yet cognitive science tells us that perception develops in the context of acting and moving in the world---and without intensive supervision. How can unlabeled video augment computational visual learning? I’ll overview our work exploring how a system can learn effective representations by watching unlabeled video. First we consider how the ego-motion signals accompanying a video provide a valuable cue during learning, allowing the system to internalize the link between “how I move” and “what I see”. Building on this link, we explore end-to-end learning for active recognition: an agent learns how its motions will affect its recognition, and moves accordingly. Incorporating these ideas into various recognition tasks, we demonstrate the power in learning from ongoing, unlabeled visual observations---even overtaking traditional heavily supervised approaches in some cases.
Jim Dicarlo (Monday14:00 - 14:45)
Reverse engineering primate visual intelligence - [slides]
TBA
Karen Livescu (Monday 14:45 - 15:30)
Acoustic word embeddings
For a number of speech tasks, it can be useful to represent speech segments of arbitrary length by fixed-dimensional vectors, or embeddings. Vectors representing word segments -- acoustic word embeddings -- can be used in query-by-example search, example-based speech recognition, or spoken term discovery. *Textual* word embeddings have been common in natural language processing for a number of years now; the acoustic analogue is only recently starting to be explored. This talk will present our work on acoustic word embeddings, including a variety of models trained with different types of supervision and tested on query-by-example and related tasks.
Josh Tenembaum (Tuesday 9:00 - 9:45)
TBA
TBA
Li Fei-Fei (Tuesday 9:45 - 10:30)
TBA
TBA
Aude Oliva (Tuesday 11:00 - 11:45)
Mapping the spatio-temporal dynamics of vision in the human brain
Recognition of objects and scenes is a fundamental function of the human brain, necessitating a complex neural machinery that transforms low level visual information into semantic content. Despite significant advances in characterizing the locus and function of key visual areas, integrating the temporal and spatial dynamics of this processing stream has posed a decades-long challenge to human neuroscience. In this talk I will describe a brain mapping approach to combine magnetoencephalography (MEG), functional MRI (fMRI) measurements, and convolutional neural networks (CNN) by representational similarity analysis to yield a spatially and temporally integrated characterization of neuronal representations when observers perceive visual events. The approach is well suited to characterize the duration and sequencing of perceptual and cognitive tasks, and to place new constraints on the computational architecture of cognition.
In collaboration with: D. Pantazis, R.M Cichy, A. Torralba, S.M. Khaligh-Razavi, C. Mullin, Y. Mohsenzadeh, B.Zhou, A. Khosla
Dan Yamins (Tuesday 11:45 - 12:30)
TBA
TBA
Gabriel Kreiman (Tuesday 14:00 - 14:45)
Visual recognition in the real world: peeking inside computations the human brain - [slides]
There has been significant progress in developing biologically plausible models of visual recognition to account for rapid recognition of single objects. Vision in the real world is characterized by ubiquitous dynamic internal and external changes as well as the presence of multiple objects leading to clutter and occlusions. I will describe initial efforts to incorporate those temporal and spatial real-world constraints into visual recognition models. The talk will illustrate how invasive field potential neurophysiological recordings from the human brain help us a window to peek inside the neural machinery orchestrating visual cognition. We will provide examples that combine behavioral, physiological and computational tools to further our understanding of the mechanisms behind visual search in cluttered scenes, interpretation of dynamic movie sequences and pattern completion of heavily occluded objects. By deciphering the neural codes that underlie the spatiotemporal integrative mechanisms behind visual cognition, we can translate biological codes into artificial intelligence algorithms and solutions that exploit the robustness, efficiency, and speed of real brains.
Pat Langley (Tuesday 14:45 - 15:30)
Information-Processing Psychology, Artificial Intelligence, and the Cognitive Systems Paradigm - [slides]
In this talk, I review the role of cognitive psychology in the origins of artificial intelligence and in the latter's early progress. I examine how many key ideas about representation, performance, and learning had their inception in computational models of human cognition, and I argue that this approach to developing intelligent systems, although no longer widespread, has an important place in the field. In addition, I summarize the cognitive systems paradigm, which incorporates many of these insights, including a focus on high-level cognition, structured representations, system-level accounts, and the role of heuristics. I also claim that another psychological notion - cognitive architecture - is especially relevant to developing unified theories of the mind and integrated intelligent systems. In contrast, I argue that neuroscience, at least in its current form, has much less to offer our understanding of intelligence.
Langley, P. (2012). The cognitive systems paradigm. Advances in Cognitive Systems, 1, 3-13. http://www.cogsys.org/paper/paper-1-2/
Langley, P. (2012). Intelligent behavior in humans and machines. Advances in Cognitive Systems, 2, 3-12. http://www.cogsys.org/paper/paper-3-2-140/
Amnon Shashua (Nadav Cohen) (Tuesday 16:00 - 16:45)
Expressive Efficiency and Inductive Bias of Convolutional Networks: Analysis and Design through Hierarchical Tensor Decompositions - [slides]
The driving force behind convolutional networks - the most successful deep learning architecture to date, is their expressive power. Despite its wide acceptance and vast empirical evidence, formal analyses supporting this belief are scarce. The primary notions for formally reasoning about expressiveness are efficiency and inductive bias. Efficiency refers to the ability of a network architecture to realize functions that require an alternative architecture to be much larger. Inductive bias refers to the prioritization of some functions over others given prior knowledge regarding a task at hand. Through an equivalence to hierarchical tensor decompositions, we study the expressive efficiency and inductive bias of various architectural features in convolutional networks (depth, width, pooling geometry, inter-connectivity, overlapping operations etc). Our results shed light on the demonstrated effectiveness of convolutional networks, and in addition, provide new tools for network design.
The talk is based on a series of works done under the supervision of Prof. Amnon Shashua, with and by the students of our group: Or Sharir, Ronen Tamari, Yoav Levine and David Yakira.
Alan Yuille (Tuesday 16:45 - 17:30)
Deep Networks and Beyon - [slides]
TBA
Surya Ganguli (Wednesday 9:00 - 9:45)
Towards bridging the gap between neuroscience and artificial intelligence - [slides]
TBA
L. Mahadevan (Wednesday 9:45 - 10:30)
Geometry, Probability and Invariance in Visual Perception
I will discuss a few visual perceptual contexts that naturally link geometry and probability, motivated by the following questions: (i) what is our notion of randomness in a geometrical context ? (ii) how can we characterize geometry probabilistically to discriminate between objects? (iii) (why) do we use geometrical rules to solve problems of spatial reasoning? In each case, using theory and experiments, I will try to argue that invariances provide the key.
Panel discussion (Wednesday 11:00 - 12:30)
Biological Plausability and Theoretical Justification of Deep Learning
Moderator : Tomaso Poggio - [slides]
Panelists: David Donoho, Aude Oliva, Dan Yamins
Important Dates
Abstract submission deadline:
|
October 28, 2016
|
Acceptace Notification to Authors:
|
November 29, 2016
|
Final camera-ready abstract deadline:
|
January 31, 2017
|
Registration deadline:
|
limited number of registrations available
|
Symposium:
|
March 27-29, 2017
|
Program Chairs and Organizing Committee
Gemma Roig
(MIT)
|
Xavier Boix
(MIT)
|
gemmar@mit.edu | xboix@mit.edu |
Center for Brains, Minds and Machines
LCSL, Istituto Italiano di Tecnologia@ MIT
Massachusetts Institute of Technology
Bldg. 46-5155, 77 Massachusetts Avenue
Cambridge, MA 02139
Telf: 617-324-3684
|
Center for Brains, Minds and Machines
LCSL, Istituto Italiano di Tecnologia@ MIT
Massachusetts Institute of Technology Bldg. 46-5155, 77 Massachusetts Avenue Cambridge, MA 02139 Telf: 617-324-3684 |