fMRI Bootcamp
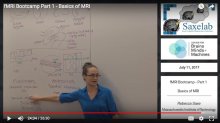
This series of nine “chalk talk” style lectures begins with an introduction to the basics of anatomical and function MRI and the time course of the fMRI signal, and then delves into several methods for analyzing fMRI data, including univariate and multivariate analyses, multivoxel pattern analysis (MVPA), representational similarity analysis, multiple comparison methods, and hyperalignment. The presentation emphasizes how to think about fMRI data and the steps of analysis, rather than elaborating the details of how to perform each step.
Taught by: Rebecca Saxe
Videos:
- fMRI Bootcamp Part 1 - Basics of fMRI (26:30) - Introduction to the basics of anatomical and functional MRI.
- fMRI Bootcamp Part 2 - fMRI timecourse (1:22:18) - Overview of the time course of the fMRI signal and its underlying physical basis: the hemodynamic response function (HRF), blood oxygen-level dependent (BOLD) signal, and relationship between the BOLD signal and the time course of the experimental stimulus conditions.
- fMRI Bootcamp Part 3 - Univariate analysis (57:52) - Review of early univariate analyses that examined the time course of fMRI signals in individual voxels to assess how much neural activity occurred in response to different stimulus conditions, and where this activity occurred, in the brains of individual subjects and across multiple people.
- fMRI Bootcamp Part 4 - Multivariate analysis (55:42) - Introduction to multivariate techniques for analyzing fMRI data, which represent and evaluate the correlation of fMRI activation across brain regions, in contrast to the voxel-by-voxel univariate analysis method, and the advantages of performing multivariate analyses.
- fMRI Bootcamp Part 5 - Multivoxel pattern analysis (MVPA) (14:25) - Continuation of the introduction to multivariate analysis techniques for fMRI data, which focuses on how and why particular voxels are selected for this analysis. For example, a certain group of voxels may be a hypothesized functional unit of analysis, or may have higher signal-to-noise characteristics that yield greater statistical power.
- fMRI Bootcamp Part 6 - Classification (35:10) - Elaboration on the classification step in fMRI analysis, in which the particular stimulus condition(s) present in a given experimental trial are learned from the observed fMRI responses of a set of voxels, enabling prediction of the stimulus condition from new data. Examples are drawn from a study by Rebecca Saxe and Amy Skerry on neural representations of emotion.
- fMRI Bootcamp Part 7 - Representational similarity (47:55) - Introduction to representational similarity analysis (RSA), which uses measures of the similarity of pairs of distributed patterns of fMRI responses to multiple stimulus conditions, in selected regions of interest (ROIs), to determine whether an experimental variable of interest is distinguished in the fMRI signals obtained in these regions.
- fMRI Bootcamp Part 8 - fMRI & multiple comparisons (1:26:13) - This tutorial addresses the problem of multiple comparisons, which arises in the analysis of fMRI data when hypothesis tests are performed over many voxels spanning a large area of the brain, in order to determine where there are significant differences in response between multiple stimulus conditions. After introducing the basic problem, a number of ways to think about the solution to the problem are elaborated.
- fMRI Bootcamp Part 9 - Hyperalignment (20:56) - Variability between people, in the precise anatomical and functional brain architectures pose a challenge for performing group analyses that integrate fMRI data across multiple subjects in fMRI experiments. This final tutorial addresses the problem of how to align the fMRI data between subjects, focussing on a method proposed by James Haxby in 2011 to perform this alignment.
Additional Resources:
- Rebecca Saxe’s Lab website
- Analyzing fMRI Data page in Modeling and Data Analysis Tools and Datasets
- Poldrack R. A., Mumford J. A., Nichols T. E. (2011) Handbook of Functional MRI Data Analysis, Cambridge University Press (hardcover, eBook)