Neural Circuits of Visual Intelligence
Overview
How does intelligent behavior emerge from neural activity in the brain? How can the analysis of computational models help us understand the underlying neural processing, and vice versa? This unit explores these questions for vision, focusing on the function of the ventral visual stream in the brains of humans and monkeys.
Videos
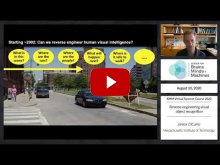
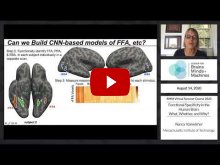
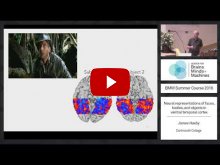
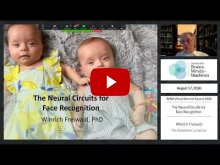
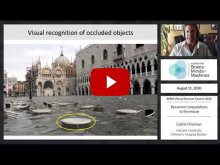
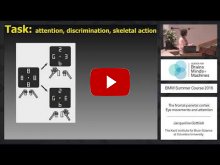
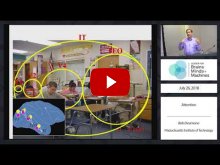
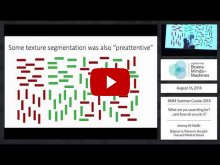
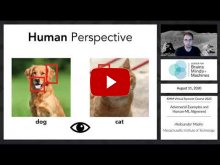
Further Study
Online Resources
Additional information about the speakers’ research and publications can be found at these websites:
- Robert Desimone (MIT)
- James DiCarlo (MIT)
- Winrich Freiwald (Rockefeller)
- Jacqueline Gottlieb (Columbia)
- James Haxby (Dartmouth)
- Nancy Kanwisher (MIT) and nancysbraintalks
- Gabriel Kreiman (Harvard)
- Aleksander Madry (MIT)
- Jeremy Wolfe (Harvard)
Also see the Brain-Score website and associated Brain-Score GitHub page, described by James DiCarlo
Nancy Kanwisher cites the following two videos that provide further background regarding functional specificity in the human brain:
- Nancy Kanwisher, Functional imaging of the human brain: A window into the organization of the human mind, Carvalho-Heineken Prize presentation at CogSci’19
- Katharina Dobs, A computational explanation for domain specificity in the human visual system, Center for Brains, Minds, and Machines Research Seminar, June, 2020
Readings
Bashivan, P., Kar, K., DiCarlo, J. J. (2019) Neural population control via deep image synthesis, Science, 364, 453
Bichot, N. P., Xu, R., Ghadooshahy, A., Williams, M. L., Desimone, R. (2019) The role of prefrontal cortex in the control of feature attention in area V4, Nature Communications, 10, 5727
Engstrom, L., Iiyas, A., Santurkar, S., Tsipras, D., Tran, B., Madry, A. (2019) Adversarial robustness as a prior for learned representations, arXiv https://arxiv.org/abs/1906.00945 (Also see posting about this paper by Engstrom et al. (2019) that appeared in gradient science)
Engstrom, L., Iiyas, A., Santurkar, S., Tsipras, D., Tran, B., Madry, A. (2019) Adversarial examples are not bugs, they are features, arXiv https://arxiv.org/abs/1905.02175 (Also see posting about this paper by Engstrom et al. (2019) that appeared in gradient science)
Freiwald, W. A., Tsao, D. Y. (2010) Functional compartmentalization and viewpoint generalization within the macaque face-processing system, Science, 330, 845-851
Gottlieb, J., Oudeyer, P.Y. (2018) Toward a neuroscience of active sampling and curiosity. Nature Reviews Neuroscience, 19(12), 758-770
Guntupalli, J. S., Hanke, M., Halchenko, Y. O., Connolly, A. C., Ramadge, P. J., Haxby, J. V. (2016) A model of representational spaces in human cortex, Cerebral Cortex, 26(6), 2919-2934
Kar, K., Kubilius, J., Schmidt, K., Issa, E. B., DiCarlo, J. J. (2019) Evidence that recurrent circuits are critical to the ventral stream’s execution of core object recognition behavior, Nature Neuroscience, 22, 974-983
Kreiman, G., Serre, T. (2020) Beyond the feedforward sweep: Feedback computations in the visual cortex, Annals of the New York Academy of Sciences, 1464(1), 222-241
Kreiman, G. (2020) Biological and Computer Vision, Cambridge University Press
Landi, S. M., Viswanathan, P., Serene, S., Freiwald, W. A. (2021) A fast link between face perception and memory in the temporal pole, Science, 373, July 1
Lee, H., Margalit, E., Jozwika, K. N., Cohen, M. A., Kanwisher, N., Yamins, D. L. K., DiCarlo, J. J. (2020) Topographic deep artificial neural networks reproduce the hallmarks of the primate inferior temporal cortex face processing network, bioRxiv doi:https://doi.org/10.1101/2020.07.09.185116
Nastase, S. A., Halchenko, Y. O., Connolly, A. C., Gobbini, M. I., Haxby, J. V. (2018) Neural responses to naturalistic clips of behaving animals in two different task contexts, Frontiers in Neuroscience, 12, 316
Ponce, C. R., Xiao, W., Schade, P. F., Hartmann, T. S., Kreiman, G., Livingstone, M. S. (2019) Evolving images for visual neurons using a deep generative network reveals coding principles and neuronal preferences, Cell, 177, 999-1009
Sha, L., Haxby, J. V., Abdi, H., Guntupalli, J. S., Oosterhof, N. N., Halchenko, Y. O., Connolly, A. C. (2015) The animacy continuum in the human ventral vision pathway, J. Cognitive Neuroscience, 27(4), 665-678
Shomstein, S., Gottlieb, J. (2016) Spatial and non-spatial aspects of visual attention: interactive cognitive mechanisms and neural underpinnings, Neuropsychologia, 92, 9-19
Tang, H., Schrimpf, M., Lotter, W., Moerman, C., Parades, A., Carob, J. O., Hardesty, W., Cox, D., Kreiman, G. (2018) Recurrent computations for visual pattern completion, Proceedings of the National Academy of Sciences, 115(35), 8835-8840
Yamins, D. L. K., Hong, H., Cadieu, C. F., Solomon, E. A., Seibert, D., DiCarlo, J. J. (2014) Performance-optimized hierarchical models predict neural responses in higher visual cortex, Proceedings of the National Academy of Sciences, 111(23), 8619-8624
Yildirim, I., Belledonne, M., Freiwald, W., Tenenbaum, J. (2020) Efficient inverse graphics in biological face processing, Science Advances, 6, eaax5979
Wolfe, J. M. (2020) Visual search: How do we find what we are looking for? Annual Review of Vision Science, 6(1), 539-562
Wolfe, J. M., Horowitz, T. S. (2017) Five factors that guide attention in visual search, Nature: Human Behavior, 1, 0058
Zhou, H., Schafer, R. J., Desimone, R. (2015) Pulvinar-cortex interactions in vision and attention, Neuron, 89, 209-220