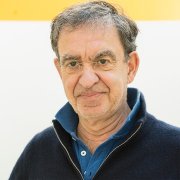
Associated Research Module:
Associated Research Thrust:
Tomaso A. Poggio, is the Eugene McDermott Professor at the Department of Brain and Cognitive Sciences; co-Director, Center for Brains, Minds and Machines; Member of the Computer Science and Artificial Intelligence Laboratory at MIT; since 2000, member of the faculty of the McGovern Institute for Brain Research.
Born in Genoa, Italy (naturalized in 1994), he received his Doctor in Theoretical Physics from the University of Genoa in 1971 and was a Wissenschaftlicher Assistant, Max Planck Institut für Biologische Kybernetik, Tüebingen, Germany from 1972 until 1981 when he became Associate Professor at MIT. He is an honorary member of the Neuroscience Research Program, a member of the American Academy of Arts and Sciences and a Founding Fellow of AAAI.
He received several awards such as the Otto-Hahn-Medaille Award of the Max-Planck-Society, the Max Planck Research Award (with M. Fahle), from the Alexander von Humboldt Foundation, the MIT 50K Entrepreneurship Competition Award, the Laurea Honoris Causa from the University of Pavia in 2000 (Volta Bicentennial), the 2003 Gabor Award, the 2009 Okawa prize, the American Association for the Advancement of Science (AAAS) Fellowship (2009), the 2017 Rosenfeld Lifetime award, won the first edition of the international Scientific Award "Ratio et Spes" in 2020, the Swartz Prize for Theoretical and Computational Neuroscience in 2014, the ICCV 2021 Helmhltz Prize and the IMPU 2022 Kampe’ de F’eriet Award and Plenary Lecture.
He is one of the most cited computational neuroscientists (with a h-index greater than 100 – based on GoogleScholar). A former Corporate Fellow of Thinking Machines Corporation and a former director of PHZ Capital Partners, Inc., is a director of Mobileye and was involved in starting, or investing in, several other high tech companies including Arris Pharmaceutical, nFX, Imagen, Digital Persona and Deep Mind. Among his PhD students and post-docs are some of the today’s leaders in the Science and in the Engineering of Intelligence, from Christof Koch (President and Chief Scientific Officer, Allen Institute) to Amnon Shashua (CTO and founder, Mobileye) and Demis Hassabis (CEO and founder, Deep Mind).